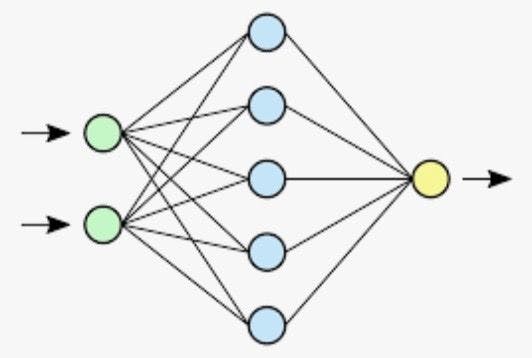
Neural nets, a type of machine learning loosely inspired by the way biological neurons connect, represent the most powerful form of machine learning. The application of neural nets to language involves setting up simple (for humans) language tasks such as “guess the missing word.” The neural net starts in a random state and its guesses are laughably bad. But through a massive amount of trial and error and self-correction, the neural net “trains” itself to more accurately predict missing words.